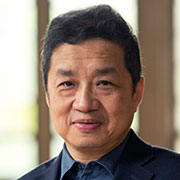
With video conferencing platforms such as Zoom experiencing hundreds of millions of users each day, Jie Wu has developed a technique to defend against real-time deepfake video chat forgeries.
Deepfake (a portmanteau of “deep learning” and “fake”) forgeries use artificial intelligence and machine learning techniques to superimpose existing images and videos onto another subject’s face—techniques that have been used to create fake celebrity pornographic videos, bogus news and malicious hoaxes.
“The problem is, how do you know the person you are talking to is a real person?” asks Wu, Laura H. Carnell Professor of Computer Engineering, director of the Temple Center for Networked Computing and AAAS and IEEE Fellow.
In a paper published in the Proceedings of the 40th International Conference on Distributed Computing Systems, Wu and one of his former doctoral students, Jiacheng Shang, describe a technique they developed that requires nothing more than the camera and screen found on most computers. Their solution involves using the screen to emit beams of light towards the supposed person while the computer’s camera senses the light reflected off that person’s face.
“With legitimate users, the light reflected off their face is proportional to the screen light,” says Wu. “Since face reenactment attackers cannot generate the real-time face reflection in a photo-realistic fashion, legitimate users can detect the face forgery.” Experimental results indicate the technology flags fakes with nearly 95 percent mean accuracy. This research has been funded, in part, by several grants from the National Science Foundation, Army Research Office and Office of Naval Research.